Around the globe, the number of connected devices forming the Internet of Things (IoT) is growing rapidly, with current projections predicting the total fleet of IoT devices will double — from 15.1 billion in 2023 to 29 billion — before the end of the decade. As devices proliferate, organizations increasingly rely on IoT analytics to guide decisions and strategies.
However, extracting accurate, reliable data analytics from IoT data feeds is a complex task with many potential pitfalls for data quality. This guide examines the major challenges associated with achieving high-quality IoT analytics and actionable steps your organization can take to overcome them.
Key Takeaways
- The rapid proliferation of IoT devices globally demands sophisticated analytics, but extracting accurate data presents multifaceted challenges.
- Achieving high-quality IoT analytics requires addressing issues like data volume, unification, scalability, compatibility, and modern versus traditional integration methods.
- Implementing comprehensive integration strategies, setting data standards, modernizing systems, and leveraging current integration platforms are pivotal to overcoming IoT data challenges.
Want to Build Your Own Mobile App?
How Businesses Use IoT Data for Analytics
Real-world applications for IoT data include:
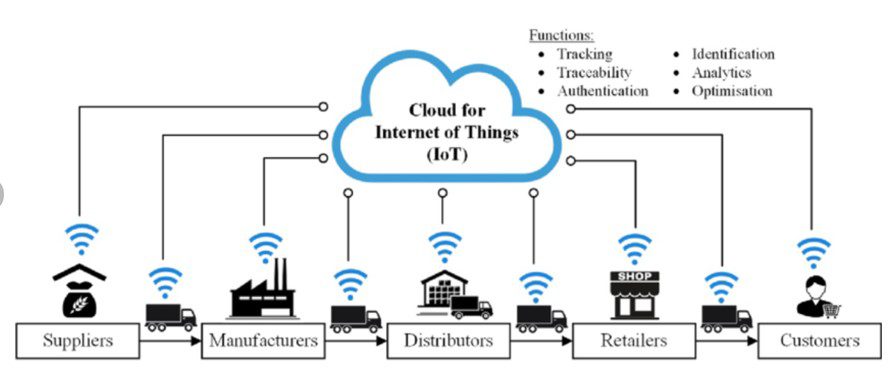
- Supply Chain and Inventory Management: Real-time tracking of goods and assets streamlines inventory processes. By embedding sensors in products, businesses can monitor stock levels, predict demand, and automate reordering to reduce overhead costs.
- Smart Facilities and Energy Management: Many enterprises employ IoT-enabled building management systems to monitor and control energy consumption, thereby reducing operational costs and contributing to sustainability objectives.
- Fleet Management: Telematics solutions, empowered by IoT, allow businesses to track vehicles in real time, monitor driver behavior, and optimize routes, resulting in enhanced efficiency and reduced fuel costs.
- Remote Monitoring and Maintenance: Manufacturers use IoT sensors to predict equipment failures, schedule proactive maintenance, and minimize downtime.
- Personalized Marketing and Retail Solutions: Retailers harness IoT technology for personalized marketing initiatives, using data from customer behaviors to tailor promotions, optimize store layouts, and enhance the overall shopping experience.
- Health and Safety Monitoring: By integrating IoT sensors in workplaces, businesses can ensure real-time monitoring of environmental conditions, detect hazardous situations, and promptly alert employees, thus fostering a safer work environment.
Challenges in IoT Data Quality and Analytics
Organizing and interpreting IoT data for practical use is a complex task for even technically savvy organizations. These are the major challenges:
1. Volume and Variety of Data
IoT devices generate vast quantities of data – a predicted 79.4 zettabytes annually by the end of 2025. Managing this influx, especially when each device possesses unique APIs, can overwhelm data management systems. The sheer diversity of unstructured or semi-structured data types, from sensor readings to camera feeds, necessitates custom solutions capable of handling different data formats and structures.
2. Data Unification Issues
Ensuring data consistency is crucial in IoT integration. Various devices may produce similar data labeled differently or datasets that don’t correspond with others in the way that human users expect them to. Harmonizing this diverse information and avoiding data duplications and disparities is pivotal for extracting meaningful insights from the data.
3. Scalability Concerns
As the number of IoT devices in an organization grows, so does the strain on network resources and databases. Increased network traffic demands more bandwidth, while the volume of data requires augmented storage solutions. Forward-thinking strategies are essential to anticipate and cater to these escalating needs without compromising performance or data integrity.
4. Compatibility and Standardization
IoT technologies often come from a variety of vendors, each adhering to different standards and communication protocols. These disparities complicate data centralization efforts. Aligning diverse technological standards and ensuring data quality and reliability becomes a complex endeavor in multi-vendor environments.
5. Traditional Versus Modern Integration Methods
Legacy systems, designed for batch processing, often falter when faced with live data streams from IoT devices. These systems might lack the requisite latency tolerance and often can’t accommodate the diverse communication protocols newer IoT devices employ. Thus, modern integration demands transcend the capacities of many traditional platforms.
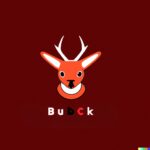
Mark hendry
CTO and Co-Founder, CMM
Nidhi is a freelance content writer with an experience of over 4 years. She uses a strategic process to come of over 4 years. She uses a strategic process to come of
Strategies to Overcome IoT Challenges
These five strategies provide a practical plan for achieving high-quality IoT analytics:
1. Develop a Comprehensive Data Integration Strategy
Begin by comprehensively mapping out your organization’s integration requirements. Understand the data sources you currently employ and determine which systems and equipment you aim to gather data from in the future. By identifying all touchpoints in your data pipeline, you can formulate a strategy to centralize and harmonize your data sources.
2. Establish Data Quality Standards
Data integrity remains paramount. Assemble a dedicated team to draft documented data quality criteria. This team should outline data formats, structures, and delivery methods that seamlessly align with your overarching integration strategy. With these standards in place, the chances of data disparities diminish considerably.
3. Transition Away from Legacy Systems
To stay in stride with modern IoT integration demands, consider phasing out outdated systems. Legacy platforms, particularly those reliant on batch processing, frequently cannot meet the rigorous data integrity and security requirements of IoT. Investing in state-of-the-art systems not only elevates data management capabilities but also conserves resources otherwise expended in maintaining obsolete platforms.
4. Adapt to IoT Communication Protocols
Familiarize yourself with the various communication protocols IoT devices utilize. While some organizations may standardize on a single protocol, others might need to cater to multiple communication methods. Ensure your chosen protocols align with your data integration strategy and consider potential network upgrades to accommodate data transmission needs.
5. Leverage Modern Integration Platforms
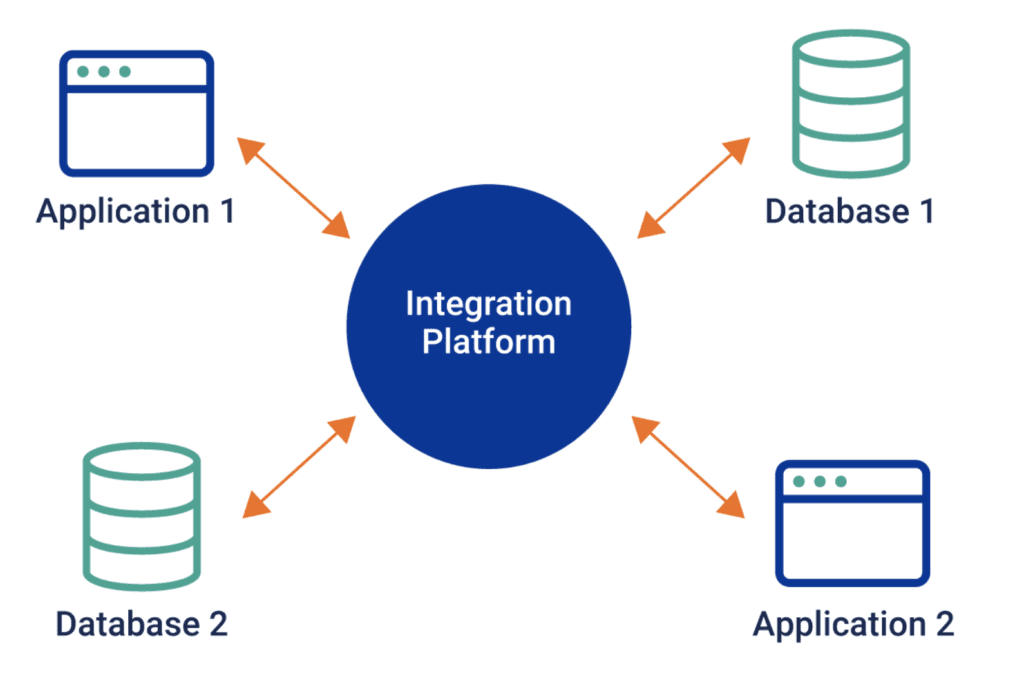
Modern data integration platforms, such as Integration-Platform-as-a-Service (IPaaS) solutions, provide robust tools to address contemporary IoT challenges. These platforms support state-of-the-art data integration practices like data virtualization and edge computing. By leveraging these solutions, you can offload much of the integration workload from your IT department, enabling a more efficient and streamlined data management process.
Extract High-Quality Data from Diverse Sources with DataBuck from FirstEigen
FirstEigen’s DataBuck leverages machine learning to measure data trustability and enable autonomous data quality validations in complex, high-volume data management environments. With DataBuck, your organization can reconcile complex data across multiple platforms automatically in an intuitive, no-code UI.
To learn more and schedule a demo, contact FirstEigen today.
Check out these articles on Data Trustability, Observability, and Data Quality.