Informatica Data Quality (IDQ): Pros, Cons, and Alternatives
Many enterprises worldwide rely on IDQ to monitor and manage the quality of data they use for day-to-day operations. IDQ – one of the oldest data quality management solutions available today – provides a platform for data analysis and data quality validation by writing rules.
Although IDQ is a popular and trusted data quality tool, it may not be the best solution for every organization. This article describes how it works, its pros and cons, and alternatives to consider.
Quick Takeaways
- Informatica Data Quality (IDQ) is a suite of tools for managing data quality.
- The solution has two primary components: Data Quality Workbench and Data Quality Server.
- IDQ improves
- Business intelligence and analytics
- Supports data governance and compliance, and
- Allows to create intelligent processes with some pre-built data accelerators.
- IDQ’s primary drawbacks include
- High license cost
- Hefty hardware requirements
- Long and difficult installation and upgrade processes
- Challenges in integrating with third-party applications
- Extremely laborious process of creating (static) DQ rules
- Resource intensive to maintain and update DQ rules
- An outdated user interface
- Not preferred for cloud use cases
- FirstEigen’s DataBuck is a better alternative to IDQ
- It complements IDQ
- It provides more automation with enhanced productivity and scalability
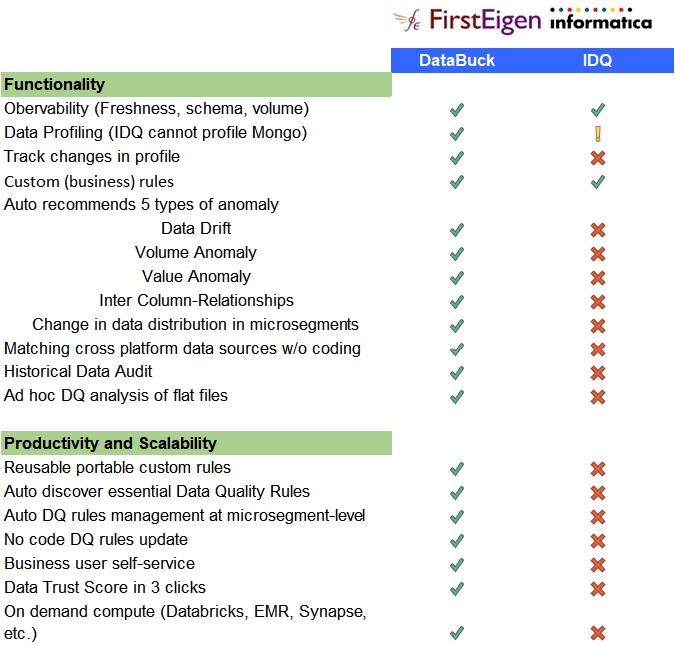
Informatica users get a free autonomous data validation add-on
What is Informatica Data Quality?
Informatica Data Quality, also known as Informatica DQ or IDQ or CDQ (for Cloud), is a suite of tools for optimizing data quality. Available in both on-premises and cloud versions, it’s targeted at enterprise users for identifying and cleaning inaccurate, corrupted, or incomplete data.
Informatica, the company, offers a variety of data-related tools, including solutions for:
- Data quality
- Data management
- Data cataloging
- Data integration
- Data governance
Founded in 1993, the company claims revenues over $1.5 billion and more than 9,500 clients for its various data management solutions. Informatica is listed on the New York Stock Exchange under the symbol INFA.
Key Components of Informatica DQ
IDQ consists of two primary components: Data Quality Workbench and Data Quality Server. The two components work together to identify and clean poor-quality data and then re-integrate that data into a company’s data stream.
Data Quality Workbench
This enables users to design, test, and ultimately deploy data quality processes, which Informatica calls plans. A plan is a self-contained collection of data process components, including:
- Data sources for input data
- Data sinks for data output
- Operational components for basic data analysis and enhancement
IDQ provides 50 data components, including pre-built rules and accelerators, for use in developing new plans. The rules and accelerators can also automate plans. You can easily reuse the rules and procedures you create in one plan in other plans. In addition, data managers and analysts can use Workbench’s browser-based Data Quality Analyst Tool to create custom data quality rules.
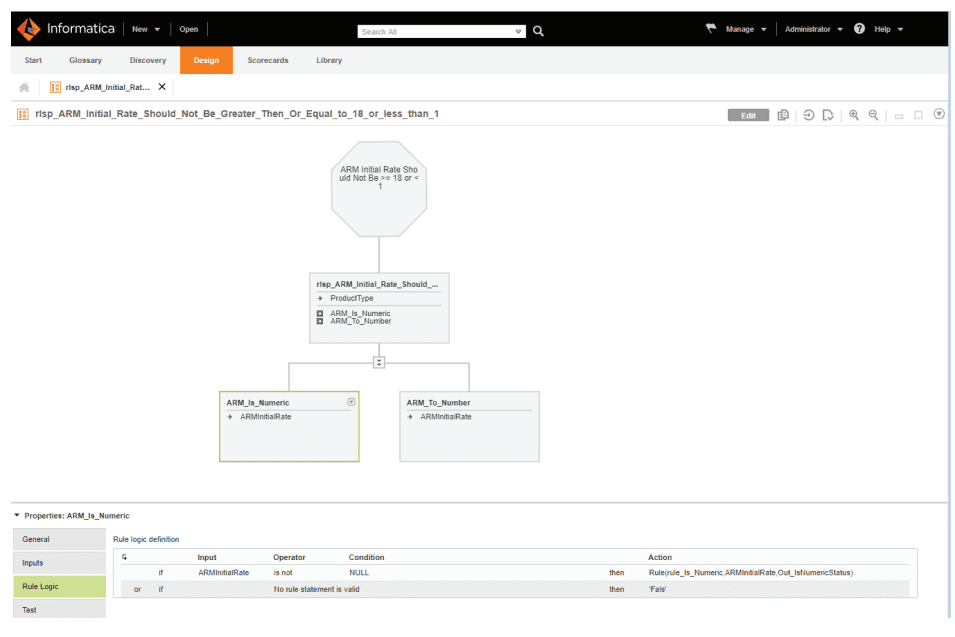
Data Quality Server
Enterprises use Data Quality Server to run data quality plans across a network. It enables both file and plan sharing and communicates with Data Quality Workbench via TCP/IP.
How Informatica Data Quality Works
In addition to Data Quality Workbench and Data Quality Server, IDQ has a data quality engine (for running plans) and a data quality repository (for storing plans). Users can apply parameter files to modify plan operations in a runtime environment.
The IDQ architecture enables three key operations: data profiling, data monitoring, and data analysis.
Data Profiling
Profiling makes complex data more accessible by delineating the behavior of individual data columns. This helps the Subject Matter Experts (SMEs) to understand the behavior of data and allows them to create data validation rules that can be coded by the IT team ensure inaccurate data is isolated.
Data Monitoring
Data monitoring involves analyzing incoming data with a set of predetermined static DQ rules and identifying data that does not meet quality standards. Data is monitored for accuracy, completeness, consistency, timeliness, uniqueness, and validity. Data that does not meet quality standards is either removed or cleaned. IDQ’s data monitoring functionality offers various statistics and reports designed to draw attention to potential data quality issues.
CAVEAT – Since data from markets, external environments, operations, transactions, etc., are dynamic and constantly changing, the approach of using predetermined static DQ rules produces too many false positive error alerts. This causes “alert fatigue” among users.
Data Analysis
Informatica Data Quality enables detailed analysis of monitored data. IDQ includes unique data visualizations, dashboards, and reports that help you extract actionable insights from your data. This makes it easy to identify important trends and patterns, informing operational and strategic business decisions.
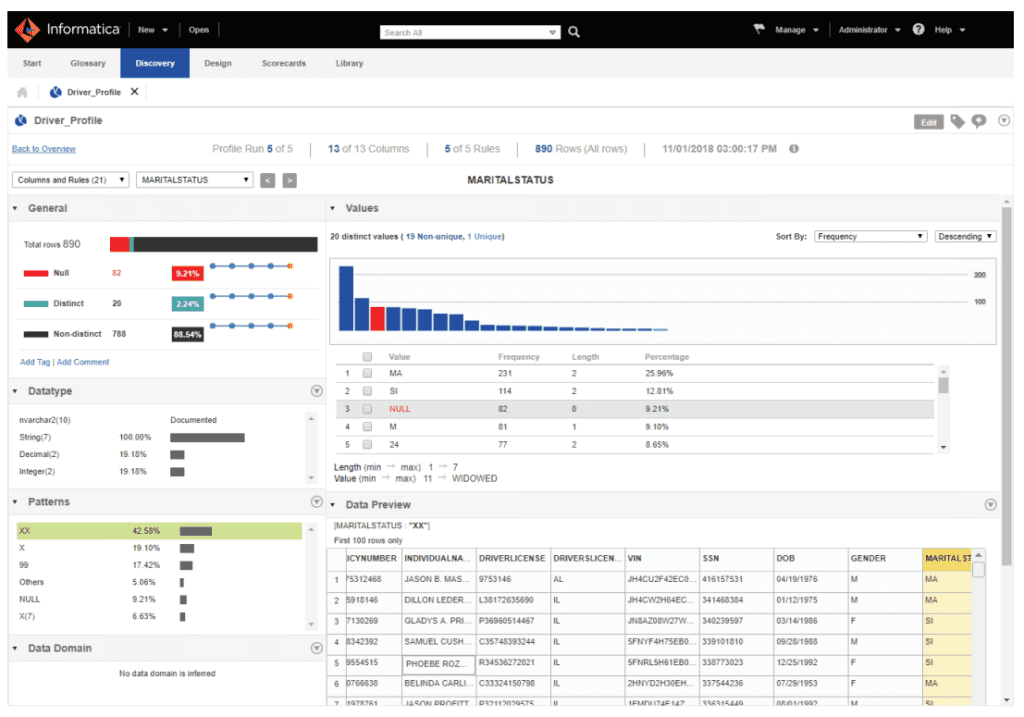
Pros and Cons of IDQ
IDQ has pros and cons. Its position as an older, more established data quality solution contributes both to its reliable results and somewhat-dated interface and operation.
IDQ’s Primary Benefits
Users choose Informatica Data Quality for various reasons, including:
- Can be bought as a part of Informatica ETL package
- Improves business intelligence and analytics
- Provides a platform for high-quality data
- Data governance and compliance support
- User-friendly drag-and-drop interface
- Few pre-built data accelerators
- On-premises and cloud availability
IDQ’s Main Drawbacks
Informatica Data Quality has a large base of satisfied users, but it’s not without its drawbacks. Users cite various issues with IDQ, including:
- Expensive
- Not designed for smaller organizations
- Significant hardware requirements
- Outdated user interface
- Difficult upgrade process
- Slow, sometimes unresponsive technical support
- Integration challenges with third-party applications
- Limited features in the cloud version compared to the on-premises version
- Extremely laborious process of understanding data and creating (static) data validation rules
- Requiring an army of enginers to maintain and update DQ rules to reflect dynamic data
These drawbacks make IDQ a less-than-ideal solution for smaller and mid-sized businesses, as well as companies that need to integrate data with third-party solutions or for cloud use cases.
Alternatives to Informatica Data Quality
If IDQ isn’t the right solution for your organization, consider these competitors in the data quality space:
- Ataccama ONE Platform
- DataBuck
- Egnyte
- Experian Aperture Data Studio
- IBM InfoSphere QualityStage
- Microsoft Purview
- Oracle Enterprise Data Quality
- SAP Data Services
- Talend Open Study for Data Quality
Of these alternatives, DataBuck’s Data Quality and Data Trustability Modules provide autonomous DQ solutions that don’t require manual coding. Results integrate directly into most third-party data governance tools, including Informatica Axon, Microsoft Purview, Alation, etc.
FirstEigen’s DataBuck: A Modern Data Quality Tool for Businesses of All Sizes
Whether you’re a Fortune 100 company or a single-location operation, FirstEigen’s DataBuck can help you optimize the quality of your data. DataBuck is a modern data quality tool that uses artificial intelligence and machine learning technologies to quickly and accurately validate the quality of both internal and ingested data. DataBuck is fast, cost-effective, and easily scalable.
Contact FirstEigen today to learn more about improving the quality of your organization’s data.
Check out these articles on Data Trustability, Observability, and Data Quality.