Previously published in Entrepreneur.com
You’ve finally moved to the Cloud. Congratulations! But now that your data is in the Cloud, can you trust it? With more and more applications moving to the cloud, the quality of data is becoming a growing concern. Erroneous data can cause all sorts of problems for businesses, including decreased efficiency, lost revenue, and even compliance issues. This blog post will discuss the causes of poor data quality and what companies can do to improve it.
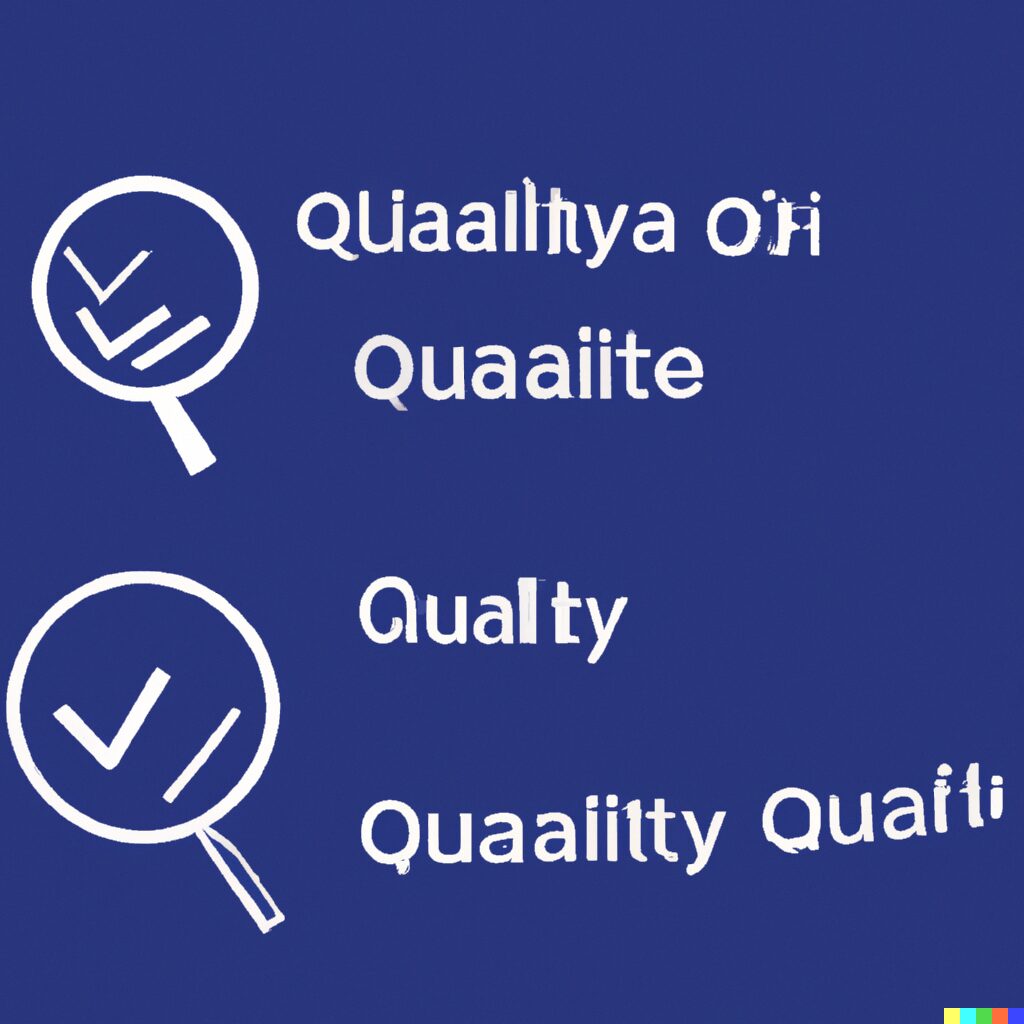
Ensuring data quality has always been a challenge for most enterprises. This problem increases when dealing with data in the cloud or sharing data with different external organizations because of the technical and architectural challenges. Data sharing in the cloud has become increasingly popular recently as businesses seek to take advantage of the cloud’s scalability and cost-effectiveness. However, the return on investment from these data analytics projects can be questionable without a strategy to ensure data quality.
What contributes to data quality issues in the Cloud?
Four primary factors contribute to data quality issues in the cloud:
- When you migrate your system to the cloud, the legacy data may not be of good quality. As a result, bad data gets carried forward to the new system.
- Data may become corrupted during migration, or cloud systems may not be configured correctly. For example, a Fortune 500 company restricted its cloud data warehouses to store numbers up to eight decimal points. This challenge caused truncation errors during migration resulting in a $50 million reporting issue.
- Data quality can be a problem when data from different sources need to be combined. For example, two different departments of a pharmaceutical company use different units (number versus packs) to store inventory information. When this information was combined in the cloud data warehouse, it became a nightmare to report and analyze the data because of the inconsistencies in the unit.
- Data from External Data vendors can have questionable quality.
Why validating data quality in the cloud is challenging?
Everybody knows data quality is essential. Most companies spend significant money and resources trying to improve data quality. However, despite these investments, companies lose money yearly because of bad data, ranging from $9.7 million to $14.2 million annually (https://www.entrepreneur.com/article/332238).
Traditional data quality programs do not work well for identifying data errors in cloud environments because:
- Most organizations only look at the data risks they know, which is likely only the tip of an iceberg. Usually, data quality programs focus on completeness, integrity, duplicate, and range checks. However, these checks only represent 30 to 40 percent of all data risks. Many data quality teams do not check for data drift, anomalies, or inconsistencies across sources, contributing to over 50 percent of data risks.
- The number of data sources, processes, and applications has exploded because of the rapid adoption of cloud technology, big data applications, and analytics. These data assets and processes require careful data quality control to prevent errors in downstream processes.
- The data engineering team can add hundreds of new data assets to the system in a short period. However, the data quality team usually takes about one to two weeks to put in place checks for each new data asset. This means that the data quality team has to prioritize which assets need checks first, and as a result, many assets don’t get checked at all.
- Organizational bureaucracy and red tape can often slow down data quality programs. Data is a corporate asset, so any change requires multiple approvals from different stakeholders. This can mean that data quality teams must go through a lengthy process of change requests, impact analysis, testing, and signoffs before implementing a data quality rule. This process can take weeks or even months, during which time the data may have significantly changed.
What can you do to improve the quality of the cloud data?
It is important to put a strategy in place that considers these factors to ensure data quality in the Cloud. Below are some tips for achieving data quality in the cloud:
- Check the quality of your legacy and third-party data. Fix any errors you find before migrating to the cloud. These quality checks will increase the cost and time it takes to complete the project but having a successful data environment in the cloud will be worth it.
- Reconcile the cloud data with the legacy data to ensure data was not lost or changed during the migration.
- Establish governance and control over your cloud data and process. Monitor data quality on an ongoing basis and establish corrective actions when errors are found. This will help prevent issues from getting out of hand and becoming too costly to fix.
In addition to the traditional data quality process, data quality teams must analyze and establish predictive data checks, including data drift, anomaly, data inconsistency across sources, etc. One way to achieve this is by using machine learning techniques to identify hard-to-detect data errors and augment current data quality practices. Another strategy is to adopt a more agile approach to data quality and align with the Data Operations teams to accelerate the deployment of data quality checks in the cloud.
Migrating to the cloud is complex, and data quality should be top of mind to ensure a successful transition. Adopting a strategy for achieving data quality in the cloud is essential for any business that relies on data. By considering the factors that contribute to data quality issues and putting processes and tools in place, you can ensure the highest-quality data, and your cloud data projects will have a greater chance of success.