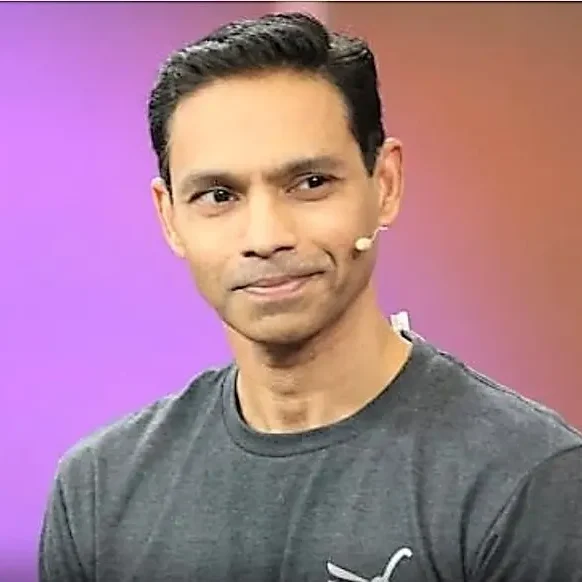
Seth Rao
CEO at FirstEigen
Understanding Data Trust: How to Enhance Data Quality and Trustworthiness with Trusted Data Formats?
Do you trust the data used by your organization? A data trust score measures how much you trust your data and is based on how accurate, up-to-date, and relevant your data is. In the end, the data trust score reflects your data’s quality—high-quality data is more trustworthy than low-quality data.
Quick Takeaways
- Organizations have to trust their data, or they won’t be able to make informed business decisions
- The data trust score measures how well a given data set can be trusted to be of high quality
- Calculate a data trust score from five key data metrics: validity, completeness, popularity, discoverability, and usage
- To improve data trust scores, improve data quality
What is Data Trust?
According to companies polled in the Talend Data Health Barometer survey, 36% say they don’t trust the data they need to make business decisions. Only 29% said they feel confident about their business decisions based on that data. Almost half said that ensuring data quality is a challenge.
Image Source: Internet
The term “data trust” reflects your confidence that a given set of data is accurate and actionable. High-quality data is more trustworthy than low-quality data and more readily usable by key staff. Data that cannot be trusted cannot be used with any degree of confidence to make key operational or strategic business decisions.
What is a Data Trust Score?
The data trust score is an attempt to quantity how trusted data is. A higher data trust score means that data is more likely to be trusted. A lower data trust score means that there are enough issues with the data to cause a lack of trust.
The data trust score is based on five key metrics:
- Data validity, which measures data quality by tracking the number of valid values in a dataset
- Data completeness, which measures the number of incomplete records in a dataset
- Data popularity, which measures a dataset’s reliability, based on certification levels and user ratings
- Data discoverability, which reflects the use of metadata (such as tags, descriptions, keywords, and the like) to make it easier to find and access specific data in a dataset
- Data usage, which tracks how often a dataset is used as a source for data pipelines
How well a dataset performs in these five areas results in the overall data trust score. This score is expressed on a scale from 0 to 5, with 5 being the highest level of trust and 0 being completely untrustworthy.
Why is a Data Trust Score Important?
Companies need to trust the data they use, although it’s understandable if they don’t. According to Experian, 77% of businesses say that inaccurate data hindered their ability to respond to COVID-related market changes. When data is limited, outdated, or unreliable, management can’t trust it—and their decision-making suffers.
One of the chief benefits of a data trust score is that it quantitatively measures the trustability of a dataset. Management no longer has to guess how much to trust given data. They can use the data trust score to determine which data earns their trust.
How Can Your Organization Improve Data Trust?
With so much data entering your system daily, how do you know which data to trust—and how can you improve overall data trustability?
Consider the following best practices to improve your organization’s data trust scores. These approaches focus on improving data quality throughout the data pipeline—the higher the data quality, the more you can trust it.
1. Recognize That It Costs More to Fix Bad Data Than It Does to Create Good Data
Some bad data can be fixed. Some can’t. But even if you can repair inaccurate or incomplete data, it’s not as cost-effective as creating or ingesting better data.
Consider the 1-10-100 rule. This rule states that you can spend $1 to improve data from the start, spend $10 to correct bad data later, or lose $100 by not fixing bad data at all. In other words, it costs less to improve data quality at the outset than to fix it later or suffer through the failures and mistakes resulting from using bad, unrepaired data.
This means you should focus your organization’s efforts on creating more accurate data and using only third-party data that meet data quality standards. Letting poor-quality data in at the outset will only cost you more later.
2. Employ Data Monitoring
Unfortunately, you probably won’t be able to create or ingest 100% perfect data. Imperfections and inaccuracies creep into even the best datasets, and you need to identify those errors before they affect your trust in the data. Use data monitoring tools such as FirstEigen’s DataBuck to monitor the data your organization creates and ingests and isolate poor-quality data for future action.
3. Clean or Delete Poor-Quality Data
What do you do with the poor-quality data you identify? The choice is three-fold. You can leave it alone and let it degrade the overall data quality and data trustability of your data. You can opt to delete bad records, which improves the resulting quality and trustability of your database even as it lowers the quantity of data. Or you can choose to repair bad data.
The latter approach improves the overall quality of your data without affecting the quantity available. There are many ways to clean poor-quality data, including:
- Comparing data values to known values in another database
- Completing incomplete fields
- Standardizing data created with different schema
- Converting unstructured data to a standardized structure
4. Add Metadata to Provide More Visibility
Metadata improves visibility, searchability, and quality. It helps you identify what’s supposed to be in a given file or dataset. If data arrives without metadata, it’s often worth the effort to add it manually. It’s certainly worth the effort to include metadata when creating new data.
5. Focus on Data Quality Metrics
One essential way to improve your data trust score is to improve your data quality, which requires focusing on six key data quality metrics:
- Accuracy
- Completeness
- Consistency
- Timeliness
- Uniqueness
- Validity
Focusing on these metrics will help you improve data quality and increase trust in your data.
Use DataBuck to Improve Your Data Trust Score
The data trust score reflects the quality of your data and how much you trust that data when making important business decisions. When you want to improve your data quality and trust score, turn to DataBuck from FirstEigen. DataBuck is an autonomous data quality management solution that automatically monitors data ingested into and flowing through your data pipeline. This results in higher-quality data—and better data trust scores.
Contact FirstEigen today to learn more about data quality and data trust scores.
Check out these articles on Data Trustability, Observability & Data Quality Management-
FAQs
Data trust refers to the confidence users have in the data they access and use, based on its accuracy, completeness, timeliness, and consistency. High data trust ensures that decisions made using the data are reliable.
A data quality score is calculated based on metrics such as accuracy, completeness, consistency, timeliness, and validity. Tools like DataBuck automate the evaluation process, offering real-time insights into the trustworthiness of your data by validating it across these dimensions.
Data trust solutions automate the processes of auditing, assessing, and cleansing data. They ensure that data is reliable, accessible, and actionable, improving business outcomes by fostering trust in the data used for analysis and decision-making.
Trusted data formats are standardized formats that ensure data consistency and integrity across different systems and platforms. These formats make it easier for businesses to ensure their data is accurate, complete, and interoperable.
Data trust encompasses more than just data quality. While data quality focuses on accuracy and consistency, data trust also includes aspects like accessibility, transparency, and reliability, ensuring that data is not only correct but also usable and available when needed.
By automating the processes of data validation, monitoring, and cleansing, data trust solutions like DataBuck ensure that only accurate and reliable data is used in decision-making, thus reducing the risk of errors and improving business efficiency.
Data trustworthiness refers to the level of confidence users have in the data's ability to accurately reflect reality and support sound decision-making. It is built through consistent data quality management and transparent data governance practices.
A data trust score helps solve challenges like ensuring the completeness, consistency, and timeliness of data across systems. It can also address issues related to duplicate or missing data, inaccuracies in reporting, and the inability to trust data for critical decision-making.
Discover How Fortune 500 Companies Use DataBuck to Cut Data Validation Costs by 50%
Recent Posts
Get Started!
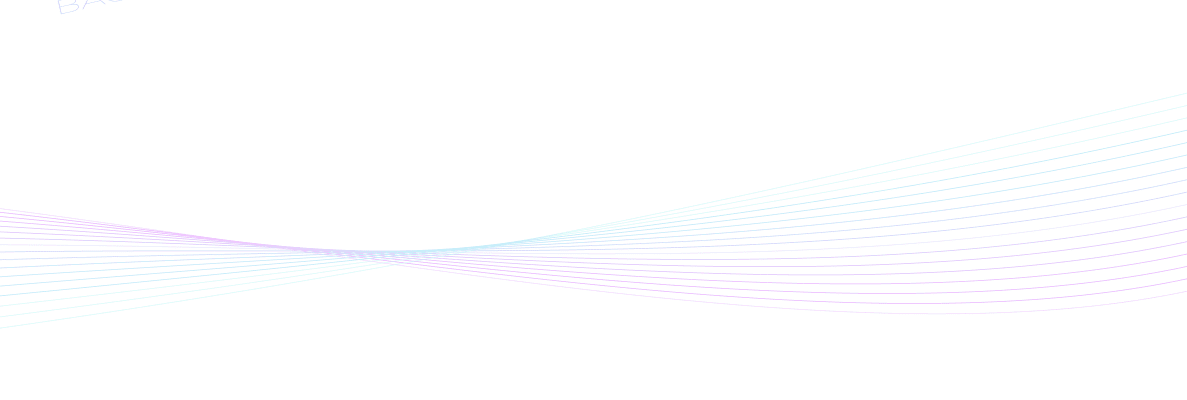