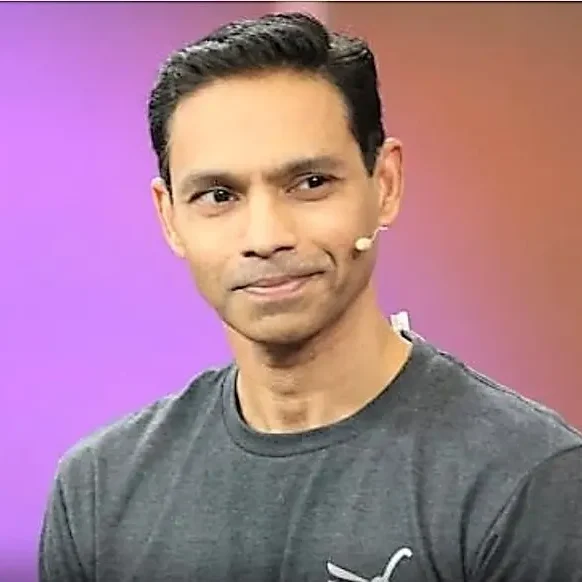
Seth Rao
CEO at FirstEigen
Data Lake vs Data Warehouse: 3 Key Differences for Effective Data Management
Picture this: You’re a chef preparing a special meal tomorrow. You have all the ingredients you need today, but now you have to decide where (and how) to store them. Do you put everything in neat, organized containers in the fridge (like a data warehouse), or do you keep them all in one big pot (like a data lake)?
Your answer will depend on the dish you’re preparing, the type of ingredients you’re working with, and the diners you’re trying to appease. Since each choice has its own unique benefits and drawbacks, the best choice will be the one that best fits your unique situation.
In the kitchen, storing ingredients may be relatively straightforward, but in the world of data, misunderstandings of the differences between data lakes and data warehouses obscure these distinctions.
Explore the three core differences between data lakes and data warehouses so that you can walk away confident in the right storage solution for your data “ingredients.”
Key Takeaways
- While data lakes and data warehouses share many similarities, they contain different types of data that must be ingested and processed differently.
- Data warehouses require a larger initial investment to structure data before ingesting it, but as a result, they’re easier to use than data lakes.
- Data lakes are easier to ingest data into, but as a result, they can require a larger recurring investment to structure data as it’s needed.
Core Difference #1: Data Structure
A data warehouse is a structured database – in other words, a database that’s well suited to storing structured data.
What is structured data? Structured data is data organized into a specific format, such as tables or columns. This type of data is relatively easy to search, analyze, and report on. Data warehouses are optimized for storing data that are already structured, making them ideal for businesses that go through a lot of structured data.
On the other hand, a data lake is an unstructured database. That doesn’t necessarily mean that all the data itself is unstructured, but just that data lakes can hold a combination of structured and unstructured data, such as text documents, social media posts, and images.
Data lakes are typically more cost efficient than data warehouses but at the expense of ease of use. Unstructured data can help businesses uncover insights that would have otherwise been invisible, but it can also be more difficult to analyze because it lacks the organization of structured data.
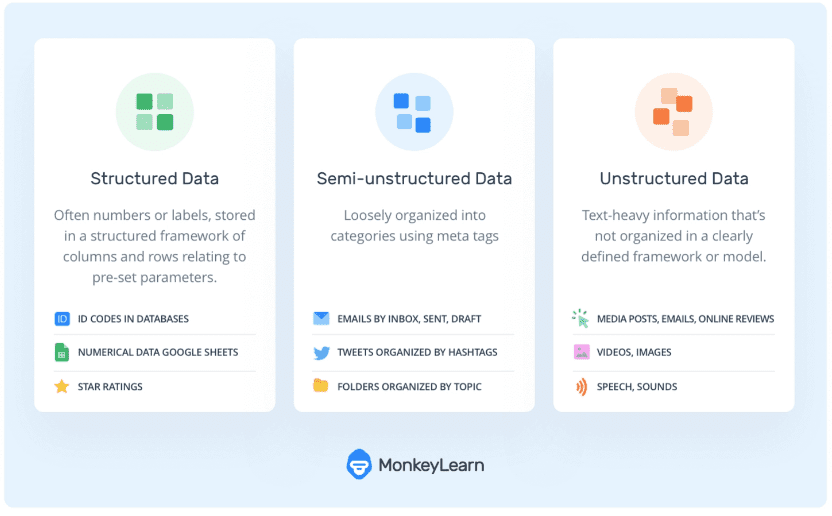
Core Difference #2: Data Ingestion
Both data lakes and data warehouses are only as good as the data they contain. The way they ingest new data is the second big difference between the two.
Data warehouses typically ingest data that’s already in a structured format. In other words, users must transform and clean data before they can load it into a data warehouse. Again, we’re faced with a give-and-take: While structured data is easier to work with, it requires an upfront investment to structure the data in the first place. This process is often time consuming and requires specialized expertise.
By contrast, data lakes are typically more flexible. They can ingest data that’s already structured or data that’s raw and unstructured. That makes data ingestion much easier for data lakes than for data warehouses, but it also means that it can create more work to transform and clean data after it’s been ingested.
In a data warehouse, users have to put more initial effort into structuring data before they can ingest it, but once it’s ingested, it’s easier to work with as a result of its structure. In a data lake, users can ingest data without putting in the work to structure it up front, but the result is that unstructured data is more difficult to draw insights from.
Core Difference #3: Data Processing
Data warehouses process data using structured queries that retrieve specific information from a structured database. This process is perfect for quickly and efficiently retrieving structured data, making it ideal for businesses that need to analyze large amounts of structured data regularly.
Since data lakes aren’t bound by the same structural constraints as a data warehouse, they’re typically more flexible in how they process data. Because the data in a data lake is unstructured, it’s compatible with a variety of tools and technologies. This allows businesses to “mix and match” structured and unstructured data to uncover insights that would be difficult to find using structured data alone.
In other words, a data warehouse is like a library where every book has a structure: each book has an author, a topic, and a book number, among others. That makes it ideal for readers who know exactly what they want. You can learn about nearly any topic if you know where to look. (After all, what is a library, if not just a warehouse for books?)
Data lakes are more like folders on your computer: They could contain structured data like a CSV file, but they could also contain images, videos, emails, social media posts, etc. This unstructured data is both a blessing and a curse. Adding a new item to a folder is much easier than adding a new book to the library, but actually making sense of the data in the folder requires more flexibility and insight.
Data Lakes vs. Data Warehouses: Which is Right for You?
When it comes to data structure, ingestion, and processing, data warehouses are less flexible but can easily find trends in structured data. By contrast, data lakes are more flexible, but that means finding trends can take extra effort.
Why does this tradeoff matter? A popular piece of advice frequently (but incorrectly) attributed to Abraham Lincoln goes like this: “If I only had an hour to chop down a tree, I’d spend the first 45 minutes sharpening my axe.”
In other words, given the choice between a difficult task that requires little preparation (chopping down a tree with a dull axe) and a relatively easier task that requires more preparation (chopping down a tree with a sharpened axe), there’s an ideal balance between ease of use and initial effort.
That ideal balance is going to be different in your company than in the company next door. There’s no one right way to store and process data, but there are options that are better suited to your unique situation.
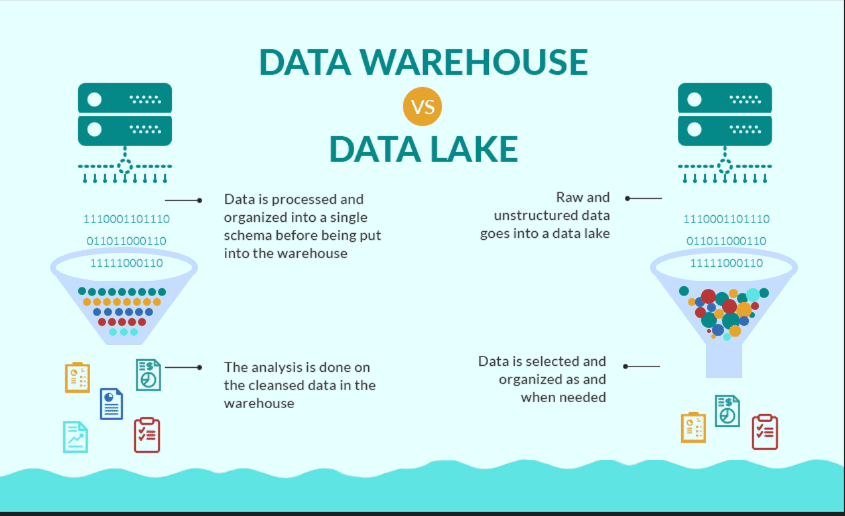
Finding Your Ideal Balance
The best data solution is the one that fits your needs.
If you’re looking for a reliable and experienced partner to help you navigate the world of data management, look no further than FirstEigen. Our team of experts has years of experience in designing, implementing, and managing data storage solutions for businesses of all sizes and industries.
We understand that every business has unique needs, and we work closely with our clients to create tailored solutions that meet their specific requirements. With our expertise in both data lakes and data warehouses, we can help you choose the right solution for your business and ensure that your data is properly stored, organized, and analyzed to drive better business outcomes.
Schedule a demo today to learn how we can help you manage your data with confidence.
Check out these articles on Data Trustability, Observability & Data Quality Management-
FAQs
Data lakes store raw, unstructured, or semi-structured data, while data warehouses store structured data optimized for analysis. Data lakes are typically more flexible but may require more effort to derive insights, while data warehouses provide faster querying for structured data but at a higher upfront cost.
A data warehouse stores structured data for fast querying, while a data lakehouse combines the flexibility of a data lake with the efficiency of a data warehouse. Databases are typically designed for transactional processing, whereas data lakes and lakehouses are built for analytics.
- Data Lakes: Scalable and cost-effective, they handle both structured and unstructured data but require more processing.
- Data Warehouses: Offer structured data storage with fast querying capabilities but come with higher setup costs.
- Data Marts: Smaller, focused subsets of data warehouses, they are quick and cost-effective for specific departments but limited in scope.
Use a data lake if you're dealing with large amounts of raw, unstructured data. A data warehouse is ideal for structured, business-critical data. A data lakehouse is suitable when you need a hybrid approach for both structured and unstructured data.
Data lakes are generally more affordable in terms of storage but may incur higher processing costs. Data warehouses are more expensive upfront but offer more efficient querying, especially for structured data.
Yes, many organizations use a hybrid approach where data lakes store raw data and data warehouses store processed, structured data. This setup allows you to benefit from both storage systems.
A data lakehouse combines the flexibility of a data lake with the structured data management of a data warehouse, making it ideal for businesses needing both raw and structured data analytics.
Data warehouses are ideal for business intelligence teams needing structured, clean data for reporting. Data lakes are better for data scientists needing unfiltered raw data for advanced analytics.
Data lakes support advanced analytics and machine learning due to their ability to store raw, unstructured data. Data warehouses, while optimized for structured data, can also integrate with AI and ML models, but are generally more rigid.
DataBuck automates the data quality and observability processes in both data lakes and warehouses. It ensures clean data ingestion, detects anomalies, and maintains data consistency, improving overall system performance.
Discover How Fortune 500 Companies Use DataBuck to Cut Data Validation Costs by 50%
Recent Posts
Get Started!
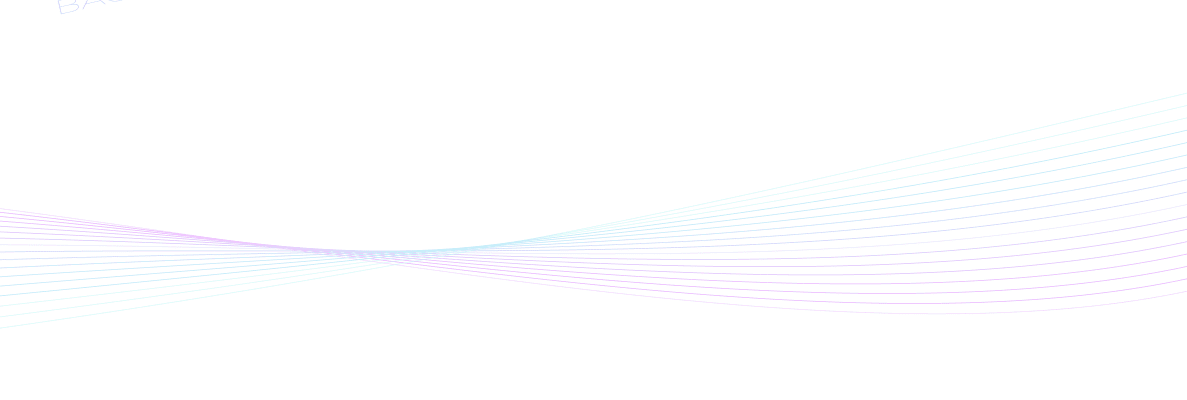