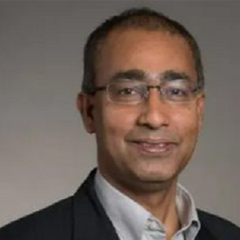
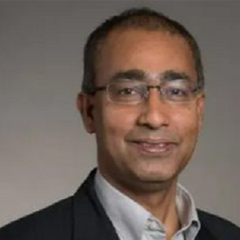
Angsuman Dutta
CTO, FirstEigen
All You Need to Know About Anomaly Detection
Maintaining data quality is important to any organization. One effective way to improve the quality of your firm’s data is to employ anomaly detection. This technique identifies data outliers that are likely to be irrelevant or inaccurate.
Understanding how anomaly detection works can help you improve your company’s overall data quality – and provide more useable data for better decision-making.
Quick Takeaways
- Anomaly detection improves data quality by identifying data points that deviate from expected patterns.
- Since outliers are likely to be poor-quality data, identifying them and isolating them improves overall data quality.
- Anomaly detection uses machine learning, artificial intelligence, and statistical algorithms to identify outlying data.
- Compared to traditional data monitoring methods, anomaly detection is more scalable and more easily handles heterogeneous data sources.
What Is a Data Anomaly?
An anomaly is a data point that does not conform to most of the data. Data anomalies are unexpected values outside the expected data pattern – they’re deviations from the norm.
Anomalies can exist in any type of data. For example, in daily sales, a day with sales twice the norm is an anomaly. In manufacturing data, a sample that is significantly bigger or smaller or heavier or lighter than the other products is an anomaly. When looking at a customer database, an 87-year-old customer among a group of 20-somethings is an anomaly.
Data anomalies are not bad but could indicate the presence of inaccuracies, miscounts, incorrect placements, or simply wrong entries. Recognizing an anomaly reveals a data point that needs to be further examined to determine its actual quality.
What is Anomaly Detection?
Anomaly detection – also known as outlier analysis – is an approach to data quality control that identifies those data points that lie outside the norms for that dataset. The thinking is that unexpected data outliers are more likely to be wrong than accurate. Truly unusual values are likely to be anomalies because something is wrong with them.
By identifying and isolating data anomalies, the remaining data – those values that conform to the expected norms – are allowed to populate the dataset. The separated, anomalous data can then be analyzed using the standard data quality metrics: accuracy, completeness, consistency, timeliness, uniqueness, and validity. If the data is determined to fail in any of these measurements, it can be deleted from the dataset or cleansed to retain its inherent value.
The key to successful anomaly detection is to first establish the normal pattern of values for a set of data and then identify data points that significantly deviate from these expected values. It’s important to not just identify the expected data values but also to specify how much deviation from these norms is considered anomalous. Advanced anomaly detection systems do this automatically using machine learning and other advanced technologies that “learn” from the data they analyze.
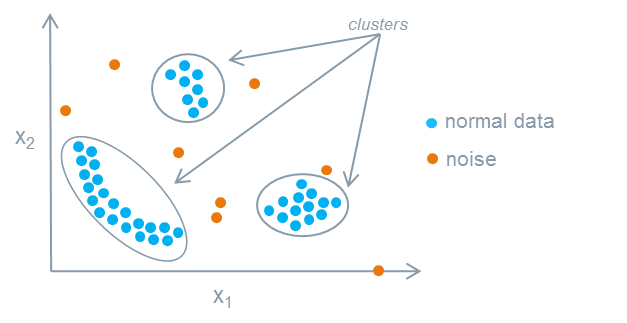
Why Is Anomaly Detection Important?
Anomaly detection is important in all industries. Whether it be in the manufacturing, financial, or sales sector, identifying potentially bad data results in a cleaner and more reliable core dataset. Eliminating anomalous data improves the overall quality and value of the data you use daily and reduces the risks of working with poor-quality or inaccurate data.
For example, in the manufacturing industry, anomaly detection is a way to improve quality control, by identifying production samples that fall outside quality standards. Anomaly detection can also help predict when individual machines require maintenance. McKinsey & Company estimates that using anomaly detection and other data-driven techniques can reduce machine downtime by up to 50% and increase machine life by up to 40%.
How Does Anomaly Detection Work?
Most anomaly detection systems use machine learning and artificial intelligence (AI) to analyze mass quantities of data, determine trends and patterns, and identify data that does not fit those patterns. These systems utilize different statistical algorithms to identify outliers in data, including:
- Robust covariance – Identifies data points lying away from the third statistical deviation.
- One class SVM – Uses support vector machine (SVM) learning technology to identify a hyperplane that best separates anomalies from the primary cluster of data points.
- One-class SVM (SGD) – Builds on the one-class SVM algorithm using Stochastic Gradient Descent (SGD).
- Isolation forest – Uses decision trees to continuously split or divide the data to eventually isolate anomalous data points.
- Local outlier factor – Examines the density of data points to determine how isolated a given data point is from the surrounding clusters.
The algorithm used often depends on the type of data being analyzed – and can produce significantly different results.
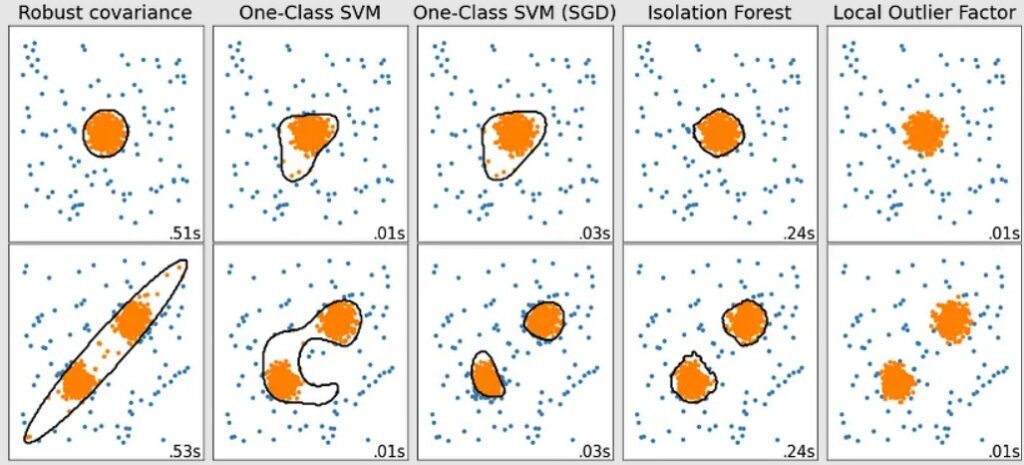
The Future of Anomaly Detection
The anomaly detection market is growing rapidly. According to the Global Anomaly Detection Industry report, the global market for anomaly detection solutions is expected to reach $8.6 billion by 2026, with a compound annual growth rate of 15.8%.
Going forward, anomaly detection will likely become more dependent on ML and AI technologies. These advanced technologies can analyze large quantities of data quickly, making them ideal for real-time monitoring of streaming data. They’re also useful for analyzing data from multiple heterogeneous sources – a task that can be challenging to perform manually. Additionally, ML/AI is more easily scalable than traditional data monitoring methods, which is important for handling the increasing growth of data facing most organizations.
Another ongoing trend in anomaly detection is the use of predictability. This involves using ML and AI technology to predict where outliers are likely to occur, allowing systems to quickly and efficiently identify anomalous data – including malicious code – before it affects data quality.
Improve Data Quality with First Eigen’s DataBuck
First Eigen’s DataBuck leverages the power of machine learning and artificial intelligence technologies to enhance data quality validation. These and other advanced technologies and algorithms identify and isolate suspicious data, automating over 70% of the data monitoring process. The result? Vastly improved data quality with minimal manual intervention.
Contact FirstEigen today to learn more about anomaly detection and data quality.
Check out these articles on Data Trustability, Observability & Data Quality Management-
Discover How Fortune 500 Companies Use DataBuck to Cut Data Validation Costs by 50%
Recent Posts
Get Start!
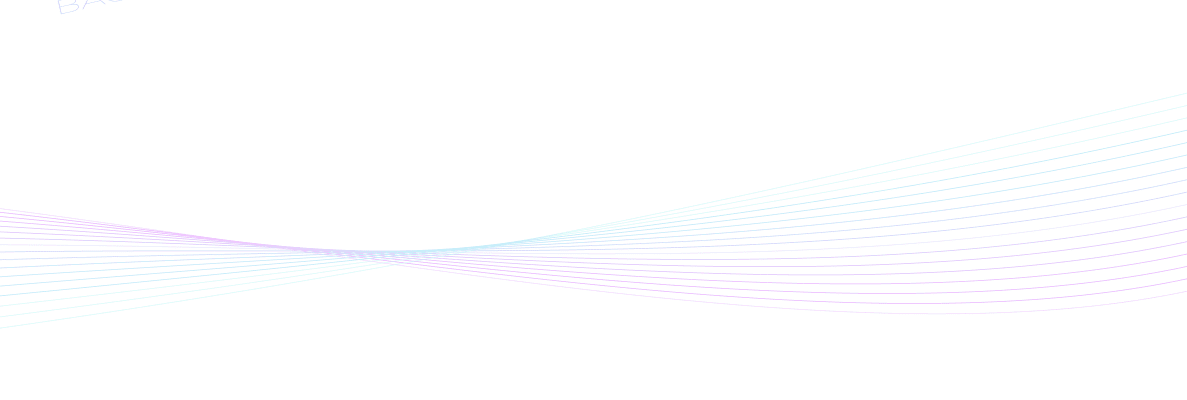
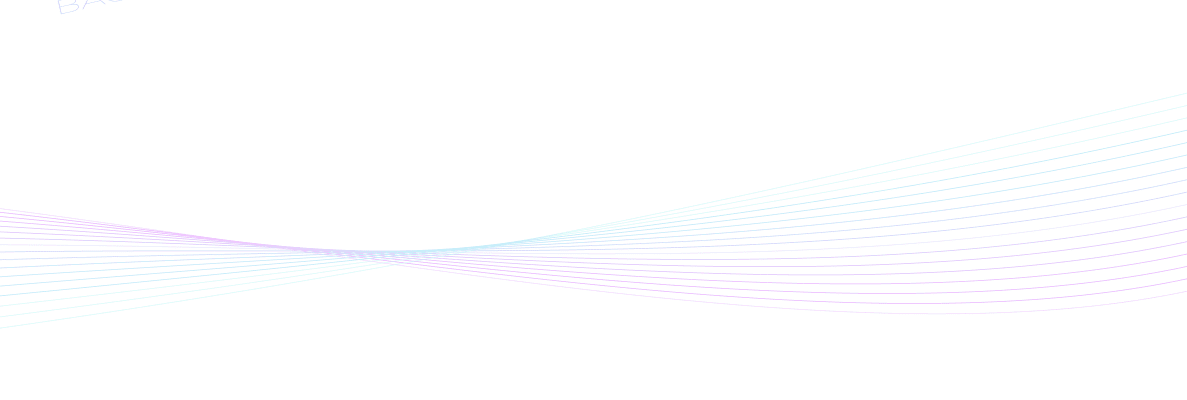