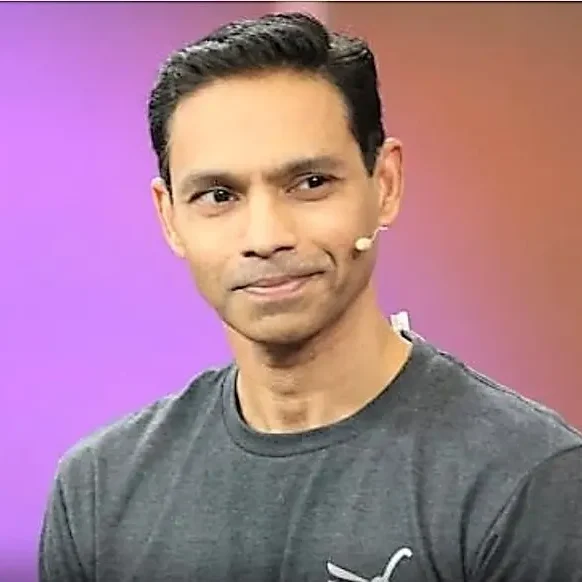
Seth Rao
CEO at FirstEigen
AI/ML-Led Automated Data Quality: a Whitepaper Overview
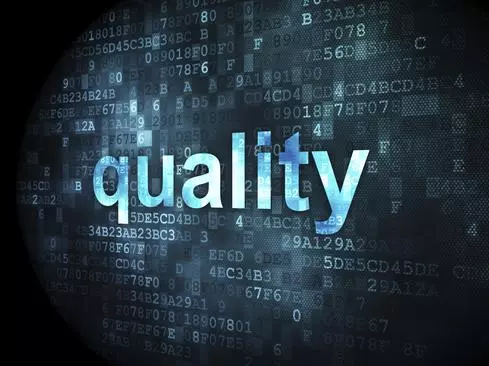
Introduction
In today’s data-driven world, Data Quality Management (DQM), especially with gen AI data quality solutions, is not just a technical requirement but a critical business imperative. Quality is one of the key functions under Data Governance, as unverified/unqualified data has little value to the organization. One of the leading global research and advisory firm estimates that an average Fortune 500 enterprise loses about $9.7mn annually over data quality issues. Although the true intangible cost of poor data is much higher, the sad truth is that data quality has not been paid the attention it deserves.
Why Traditional Data Quality Approaches Fail?
One of the reasons for this discrepancy is the way data quality issues are identified in the current systems and tools. A techno-functional team reviews data assets of an organization, and writes a set of rules to identify anomalies that are flagged for the review of data stewards. As these rules are static in nature, they become obsolete in 12- 24 months and a new assessment is required. Another significant reason is that many of the issues are contextual and are not easily codified. Consider the example of a bank that approved a corporate loan for a frequent client of theirs, at terms the client had never borrowed before, and a product that client had historically shunned. That loan should not have been approved without verifying the client’s intent. The loan data file had data quality errors; the duration of the loan was captured as 3 months and not 3 years. These subtle contextual errors cannot be caught with the traditional validation checks, like checking for completeness, uniqueness, consistency, accuracy, etc. All the checks presently done are independent of historical business context.
The Need for AI/ML-Led Data Quality Solutions
In such a dynamic business environment, the need is to augment the modernization of data management with AI-based data quality, thus achieving data semantics for delivering trusted business-critical data at organizations’ fingertips.
This is where AI/ML-led automated data quality solutions like FirstEigen’s DataBuck come in. By using AI and ML, DataBuck can autonomously discover thousands of data relationships, update validation rules as the data evolves, and monitor new data in real-time to catch unexpected errors.
Key Benefits of AI/ML-Led Automated Data Quality
- Contextual Error Detection: Unlike static rules, AI/ML-powered tools can detect subtle contextual errors that would otherwise go unnoticed.
- Continuous Learning: These systems continuously adapt, learning from data patterns and updating rules automatically as the data landscape changes.
- Real-Time Data Monitoring: AI/ML tools provide real-time validation, ensuring that data errors are caught before they can impact critical business processes.
- Reduced Manual Effort: By automating many of the repetitive tasks involved in data quality management, AI/ML reduces the manual effort required by data stewards, freeing them to focus on higher-value tasks.
To dive deeper into how AI/ML-led automated data quality can transform your organization’s approach to data governance and accuracy, download the complete whitepaper written by experts Seth Rao, Angsuman Dutta, Himansu Sekhar Tripathy, and Deep Sharma. The whitepaper discusses the challenges of traditional data quality management and explores how AI/ML technologies can significantly enhance data trust and reliability.
Download the Whitepaper Now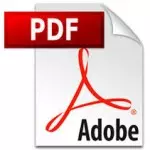
Copyright © FirstEigen, All Rights Reserved.
About the Authors
Seth Rao, Ph.D., is the CEO of FirstEigen, a Greater Chicago-based Cognitive Data Validation company. Their flagship product, DataBuck, is recognized by Gartner and IDC as the most innovative data validation software. By leveraging AI/ML it is >10x more effective in catching unexpected data errors. It increases the reliability of data by self-discovering 1,000’s of data quality relationships and patterns autonomously, updates the rules as the data evolves, and continuously monitors the new data. (https://firsteigen.com/databuck/).
Seth holds a Ph.D. in Engineering from Illinois Institute of Technology, Chicago, and has an MBA from Northwestern University’s Kellogg School of Management, USA, a BS and MS from Indian Institute of Technology, Bombay.
A. Dutta is an entrepreneur, investor and corporate strategist with experience in building software businesses that scale and drive value. In his past roles, he has provided information governance and data quality advisory services to several Fortune 500 company. He is a recognized thought leader and has published numerous articles on information governance.
He earned a Bachelor of Technology degree in engineering from the Indian Institute of Technology, Kharagpur, an MS in computer science from the Illinois Institute of Technology and an MBA in Analytical Finance and Strategy from the University of Chicago, USA.
Himansu Sekhar Tripathy is a Data Management consultant with over 18 years of experience in consulting and delivery of data solutions. His interest areas include enterprise data strategy, cloud data engineering, big data engineering, data integration, quality, metadata management, MDM, and data governance. As a technology evangelist, he believes in leveraging emerging technologies in pushing the boundaries on real time next-gen analytics. He has a master’s degree in Business Administration and a Bachelor’s degree in Computer Science Engineering.
Deep Sharma is an Associate Consultant in Cognitive & Analytics Practice unit with more than 2+ years of experience in technology consulting, analytics market research and offerings creation on emerging hybrid technology trends across the Data & Analytics technology stack. He has a keen interest in various building blocks of Data & Analytics like Data Integration, Data Quality, Data Governance and Data Visualization. He earned a Master’s Degree in Business Analytics.
How DataBuck Transforms Data Quality Management?
With DataBuck, organizations can ensure data integrity, eliminate costly errors, and drive business value through trusted data, further strengthening their AI/ML for data governance framework.
By using machine learning algorithms, DataBuck provides:
- >10x efficiency in catching unexpected data errors.
- Autonomous discovery of thousands of data quality relationships and patterns.
- Real-time monitoring of new data and automatic rule updates.
For more information about how DataBuck can help your organization achieve data quality automation, visit FirstEigen’s DataBuck.
FAQs
AI/ML-led automated data quality refers to the use of Artificial Intelligence (AI) and Machine Learning (ML) technologies to automatically detect and correct data quality issues, without relying on static rules that require frequent manual updates.
DataBuck uses AI/ML to autonomously discover data relationships, monitor changes in data in real time, and update validation rules automatically, ensuring that unforeseen errors are quickly identified and corrected.
AI/ML is critical for modern data quality management because it continuously learns and adapts to new data patterns, making it far more efficient than traditional rule-based systems, which become obsolete over time.
You can download the full whitepaper authored by Seth Rao, Angsuman Dutta, Himansu Sekhar Tripathy, and Deep Sharma by clicking here.
Discover How Fortune 500 Companies Use DataBuck to Cut Data Validation Costs by 50%
Recent Posts
Get Started!
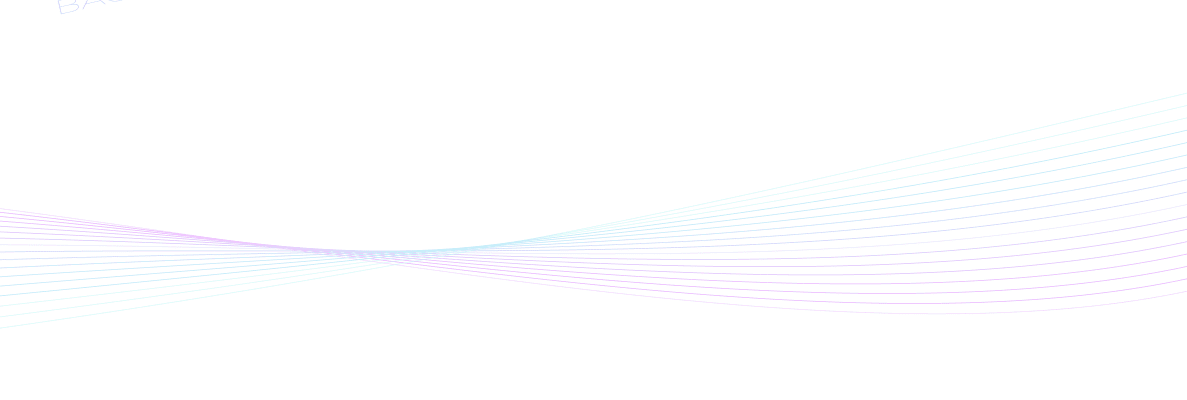