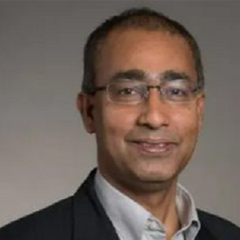
Angsuman Dutta
CTO, FirstEigen
Ditch ‘Spray and Pray’: Build Data Trust With DataBuck for Accurate Executive Reporting
In the world of modern data management, many organizations have adopted data observability solutions to improve their data quality. Initially, these solutions had a narrow focus on key areas such as detecting data freshness, schema changes, and volume fluctuations. This worked well for the early stages of data quality management, giving teams visibility into the basic health of their data pipelines.
However, as organizations scaled their use of data observability tools, the scope of these solutions expanded. The focus shifted from just monitoring the external characteristics of data to profiling the data itself. This shift, while seemingly helpful, introduced significant complexity and led to a set of new challenges that traditional observability approaches were not prepared to handle effectively.
The Overload of Automated Rules
Once data observability tools began profiling data, they started generating automated rules for each data asset. For example, a table with 150 columns might generate hundreds of rules, with each column being assessed across multiple metrics—such as completeness, conformity, consistency, and others. A typical setup could lead to 900 to 1,200 automated rule suggestions for a single data set.
This explosion in rules caused several problems:
- Resource Drain: The sheer volume of rules created a heavy review workload. Teams found themselves buried in evaluating thousands of rules and alerts, without clear guidance on which were most important. The process consumed significant resources, diverting attention from higher-priority strategic tasks.
- Overwhelming Alerts: As the number of data quality rules increased, so did the volume of alerts triggered by these rules. Data is inherently dynamic—values, formats, and volumes shift over time. This meant rules quickly became outdated, generating a flood of false positives. Teams needed larger support teams to keep up with the volume, leading to “alert fatigue.”
- Costly Compute Resources: The increased number of rules required substantial computational power to process. This led to rising costs for data validation, prompting some organizations to resort to sampling techniques. Unfortunately, sampling introduced new risks by potentially missing critical errors in unmonitored data.
The “Spray and Pray” Approach Falls Short
Many organizations have essentially adopted a “spray and pray” approach to data quality—deploying hundreds or even thousands of rules and hoping that errors are caught. However, this approach is inefficient and unsustainable. Relying on a large number of rules, especially without context or prioritization, is not the answer. In fact, it can erode confidence in data quality management altogether.
The real solution lies in precise control, where rules are deployed intelligently and dynamically, based on the actual behavior of the data. This is where DataBuck comes in, offering a more strategic, AI-driven approach to data quality management.
How DataBuck Provides a Precise Solution?
1. Leverage Machine Learning to Determine Precise Rules
Instead of generating hundreds of rules for every data set, DataBuck uses Machine Learning (ML) to evaluate each column and determine the precise rules required. It identifies whether a rule is needed based on patterns in the data and the criticality of the column, reducing the number of unnecessary checks.
2. Eliminate the Need for Sampling
Sampling introduces risk by not monitoring all data, which can lead to missed errors. DataBuck avoids this by ensuring comprehensive validation across the entire data set, without the need for risky shortcuts like sampling.
3. Move Beyond Deterministic Thresholds
Rather than relying on fixed thresholds that can quickly become outdated, DataBuck uses ML to dynamically set thresholds based on historical data patterns. This allows organizations to avoid false positives and adjust automatically as data evolves.
4. Automatically Update Rules and Thresholds as Data Changes
As data changes, so too should the rules governing its quality. DataBuck’s ML-driven engine automatically updates both rules and thresholds in response to changes in the data’s characteristics, ensuring that the validation process remains relevant and accurate over time.
5. Focus on Critical Data Elements (CDEs)
Not all data is created equal. Some data elements are more critical to the business than others, especially when it comes to executive reporting. DataBuck uses ML to identify Critical Data Elements (CDEs) and prioritize data quality rules accordingly, ensuring that the most important data is always monitored with the highest precision.
Conclusion
Data observability solutions, when initially adopted, can offer significant value. However, as organizations expand their data quality initiatives, they can easily become overwhelmed by the number of rules, alerts, and compute costs. The “spray and pray” method—where large numbers of rules are deployed in the hope that errors will be caught—simply does not work. Instead, what’s needed is precise control over data quality, with rules tailored to the specific characteristics of the data.
DataBuck provides that precision, leveraging machine learning to reduce the burden of managing data quality, while ensuring the highest levels of trust in the data used for executive reporting. By focusing on critical data elements, eliminating unnecessary rules, and using dynamic thresholds, DataBuck helps organizations manage data quality efficiently, saving time, resources, and costs.
Organizations looking to build trust in their data should adopt this targeted, ML-driven approach to ensure data integrity without the overwhelming complexity of traditional observability tools.
Discover How Fortune 500 Companies Use DataBuck to Cut Data Validation Costs by 50%
Recent Posts
Get Started!
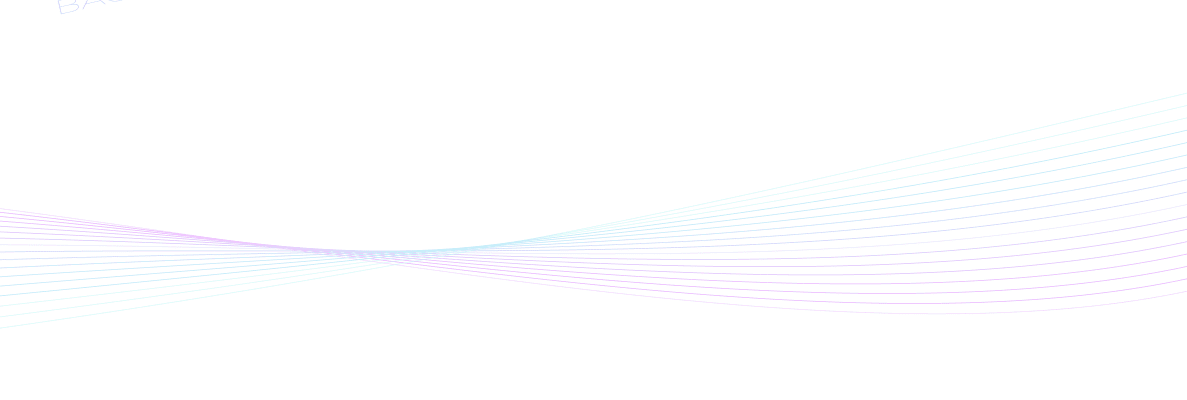