Serverless, Autonomous Data Validation in Snowflake
Ensure Superior Snowflake Data Quality With the Help of Data Trust Score
Data Quality Validation for Snowflake
Data Quality and trust are keys to making the most efficient use of data. DataBuck enables Snowflake users to evaluate Data Quality with a trust score for all data assets. DataBuck autonomously detects Data Quality specific to each dataset’s context and saves 95% of the time spent on discovering, exploring, and writing data validation rules.
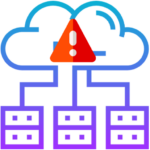
Truly-Serverless
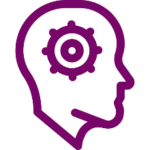
Autonomous DQ
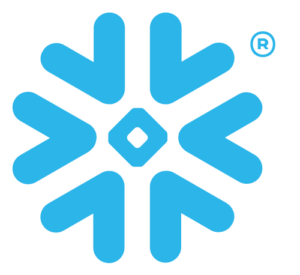
In-situ (Powered by Snowflake)
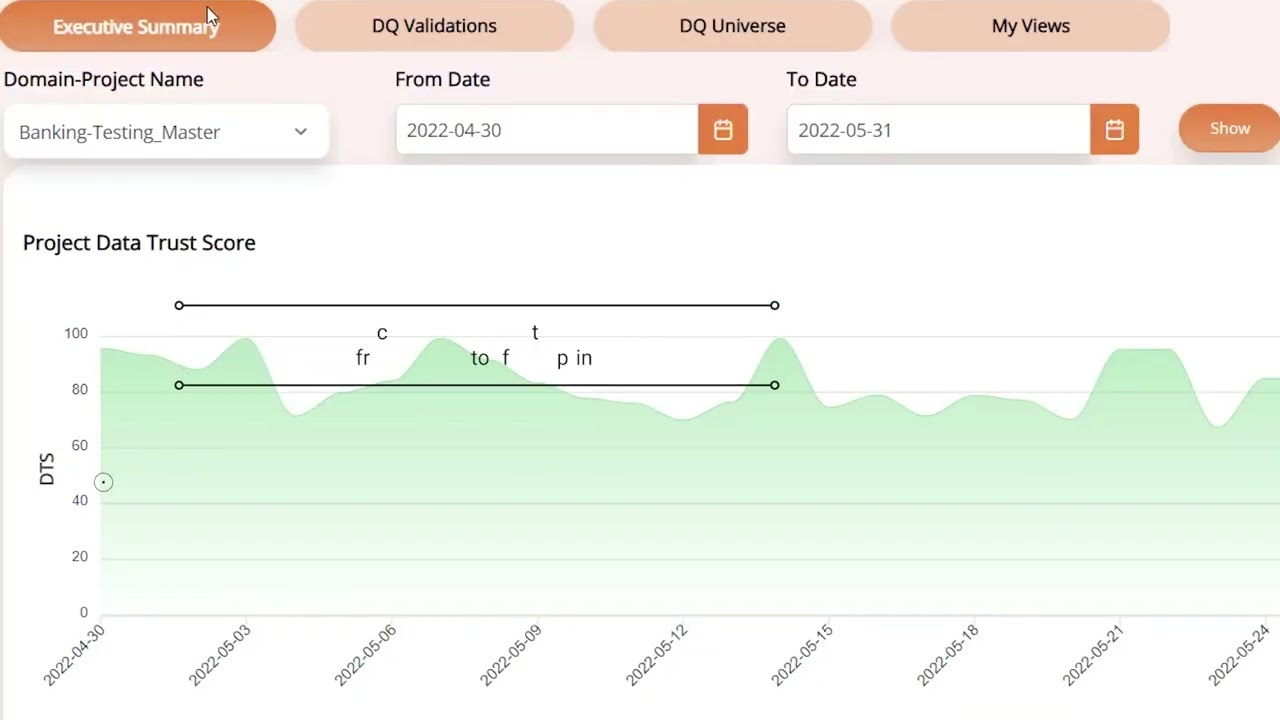
Transition from a manual model to an automated trust-based approach
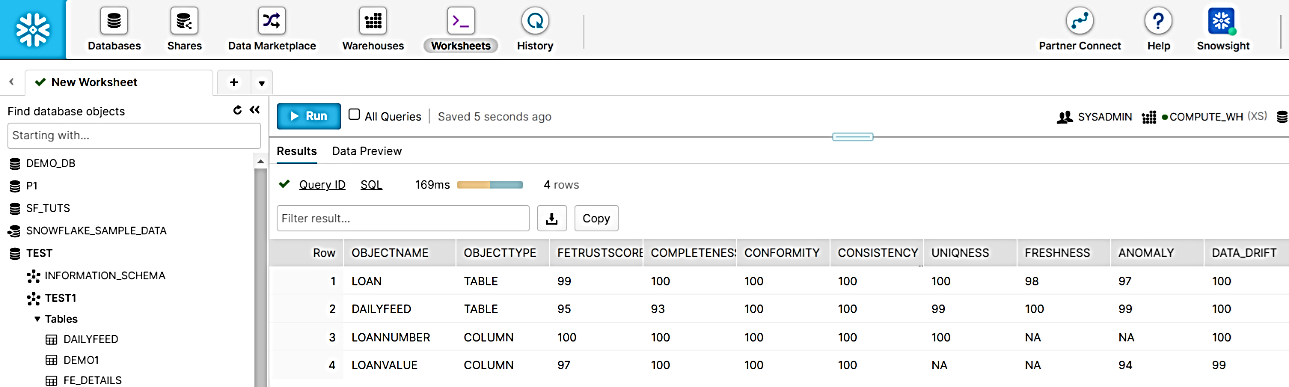
DataBuck calculates an objective Data Trust Score for each data asset (Schema, Tables, Columns) using its ML capabilities. Trust in data will no longer be a popularity contest. No need to have individuals give their subjective opinion on the health of a table/file. All stakeholders can universally understand the objective Data Trust Score. More importantly, DataBuck will map and update the data trust score to the SNOWFLAKE “OBJECT TAG” and the relevant data assets without any human intervention or complex integration efforts.
DataBuck can auto-trigger Data Trust Score as soon as new data lands in a Snowflake table or can be scheduled to run at specific time.
DataBuck can transition you from a traditional manual model to a trust-based, data-driven approach to data quality.
Know How to Establish Continuous Data Validation in Snowflake in 60 seconds
Download this white paper for more insights!
How It works
- Scan: DataBuck scans each data asset in the Snowflake platform. Assets are rescanned every time the data asset is refreshed or whenever a scheduler invokes DataBuck. Scanning is done in-situ, i.e., no data is moved to DataBuck.
- Auto Discover Metrics: DataBuck autonomously creates data health metrics specific for each data asset. The well-accepted and standardized DQ tests are customized for each data set individually, leveraging AI/ML algorithms.
- Monitor: Health metrics are computed based on quality dimensions for each column in the data asset and monitored over time to detect unacceptable data risk. Health metrics are translated to a data trust score.
- Alert: DataBuck continuously monitors the health metrics and trust score and alerts users when the trust score becomes unacceptable.
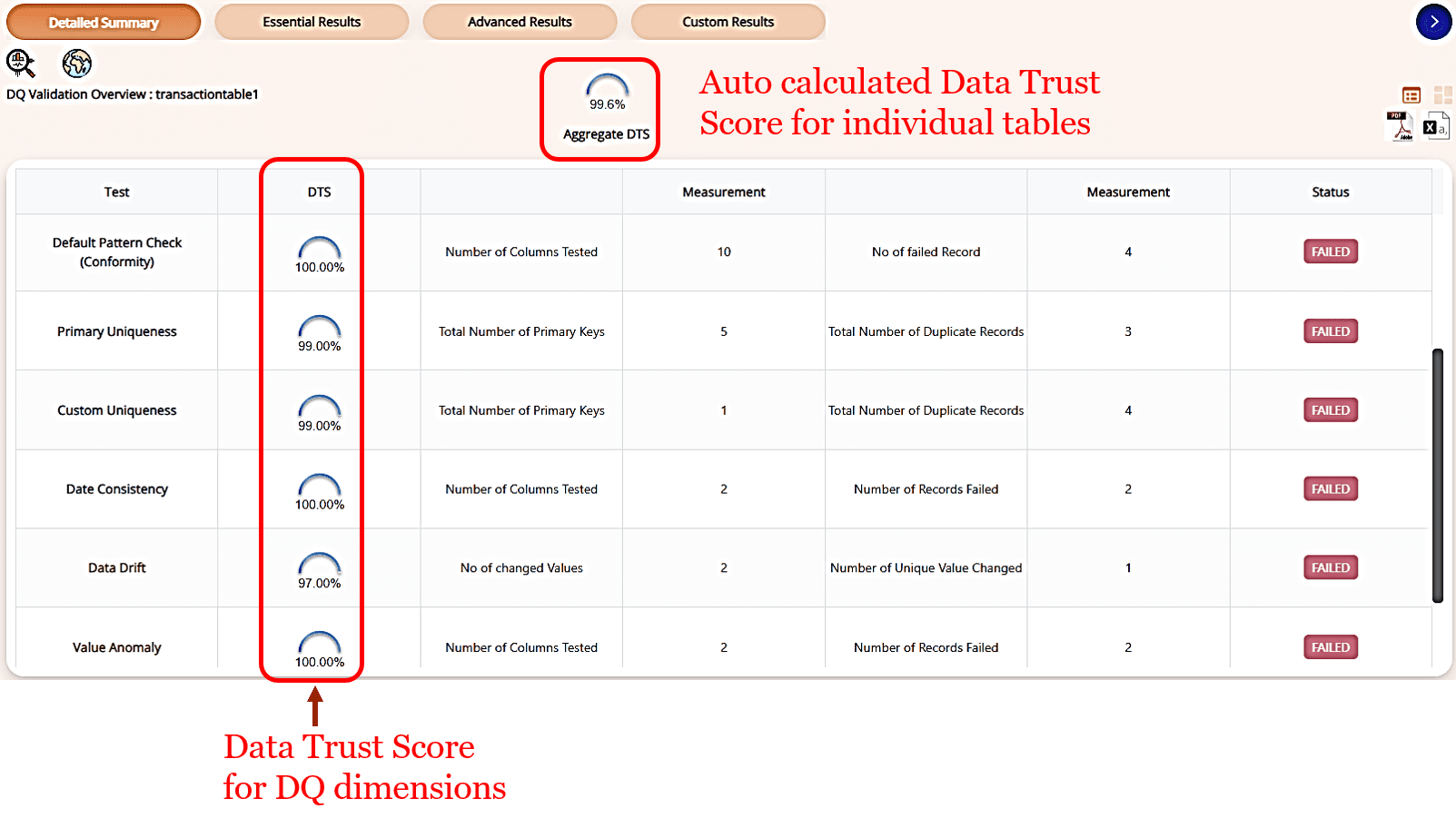
The summary of results displays the deviation in the trust score. It shows how the health and quality changed between the last two analyses and how much the user can trust the data.
Every violation discovered can be double-clicked for further information:
- Users can expand the dimension to see which columns are affected at the data asset level. Click a column name to see the dimension details for that column.
- At the column level, click the dimension name for further details.
Users can then decide whether a specific Data Quality violation can be ignored or flagged for further analysis, either for the entire data asset or individual column.
Introduction DQ Monitoring on AWS
FirstEigen recognized in AWS re:Invent as best-of-breed DQ tool
Autonomous Data Quality validation on Cloud
Friday Open House
Our development team will be available every Friday from 12:00 - 1:00 PM PT/3:00 - 4:00 PM ET. Drop by and say "Hi" to us! Click the button below for the Zoom Link: