Autonomous Data Validation in Google Cloud Platform
Ensure Superior GCP Data Quality With the Help of Data Trust Score
Mitigate the risk of incorrect data on Google Cloud Platform
Would it be useful to detect data errors upstream, so they don't get through to your business partners?
What if you could automate 80% of that work to validate data?
Cloud Data Engineers do not understand every column of every table and find it hard to validate & certify the accuracy of data. As a result, companies end up monitoring less than 5% of their data. The other 95% is unvalidated and highly risky.
DataBuck is a continuous data validation software for catching elusive data errors very early.
Powered by AI and Machine Learning, it easily integrates within your data pipeline through APIs, to discover issues for each data set and validates the reliability and accuracy of data via automation. Cut data maintenance work and cost by over 50% and certify the health of your data quality at every step of data flow automatically.
Benefit of automating data quality validation on Google Cloud Platform
Get drinkable, crystal clear stream of data from GCP along with these benefits…
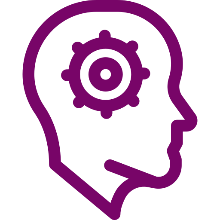
People productivity boost >80%
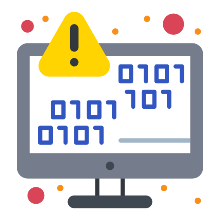
70% Reduction in unexpected errors
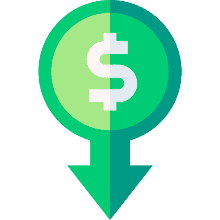
Cost reduction >50%
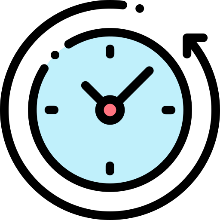
Time reduction to onboard data set ~90%
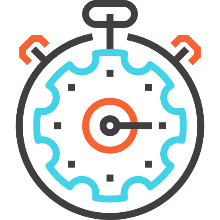
Increase in processing speed >10x

Cloud native
How It works
- Scan: DataBuck scans each data asset on the platform. Assets are rescanned every time the data asset is refreshed or whenever a scheduler invokes DataBuck. Scanning is done in-situ, i.e., no data is moved to DataBuck.
- Auto Discover Metrics: DataBuck autonomously creates data health metrics specific for each data asset. The well-accepted and standardized DQ tests are customized for each data set individually, leveraging AI/ML algorithms.
- Monitor: Health metrics are computed based on quality dimensions for each column in the data asset and monitored over time to detect unacceptable data risk. Health metrics are translated to a data trust score.
- Alert: DataBuck continuously monitors the health metrics and trust score and alerts users when the trust score becomes unacceptable.
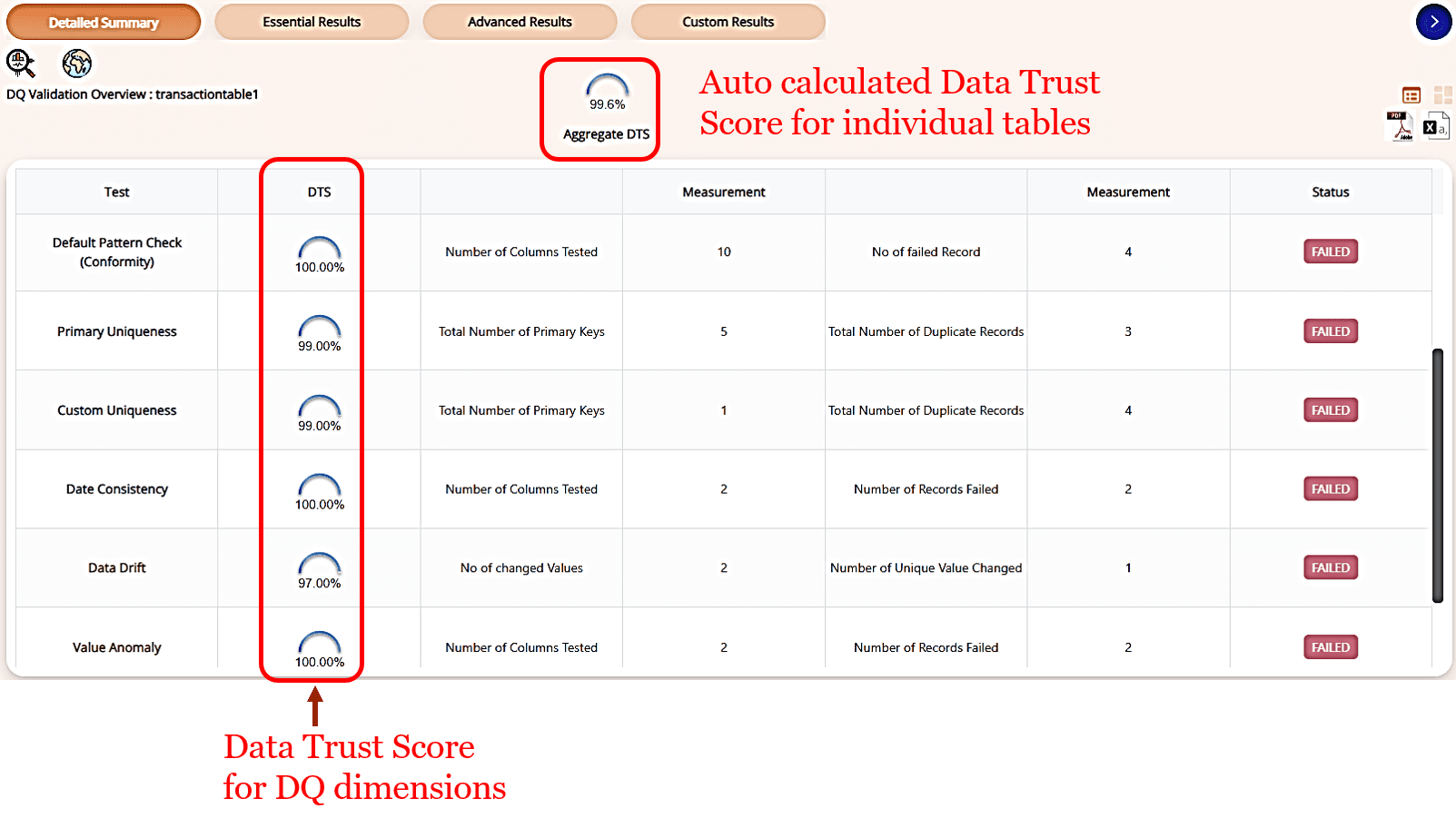
The summary of results displays the deviation in the trust score. It shows how the health and quality changed between the last two analyses and how much the user can trust the data.
Every violation discovered can be double-clicked for further information:
- Users can expand the dimension to see which columns are affected at the data asset level. Click a column name to see the dimension details for that column.
- At the column level, click the dimension name for further details.
Users can then decide whether a specific Data Quality violation can be ignored or flagged for further analysis, either for the entire data asset or individual column.
Introduction Data Quality Monitoring- Why it's important?
FirstEigen recognized in AWS re:Invent as best-of-breed DQ tool
Autonomous cloud Data Quality validation demo with DataBuck
How AI/ML simplifies Data Quality and increases accuracy
Friday Open House
Our development team will be available every Friday from 12:00 - 1:00 PM PT/3:00 - 4:00 PM ET. Drop by and say "Hi" to us! Click the button below for the Zoom Link: